AI And Behaviour Change
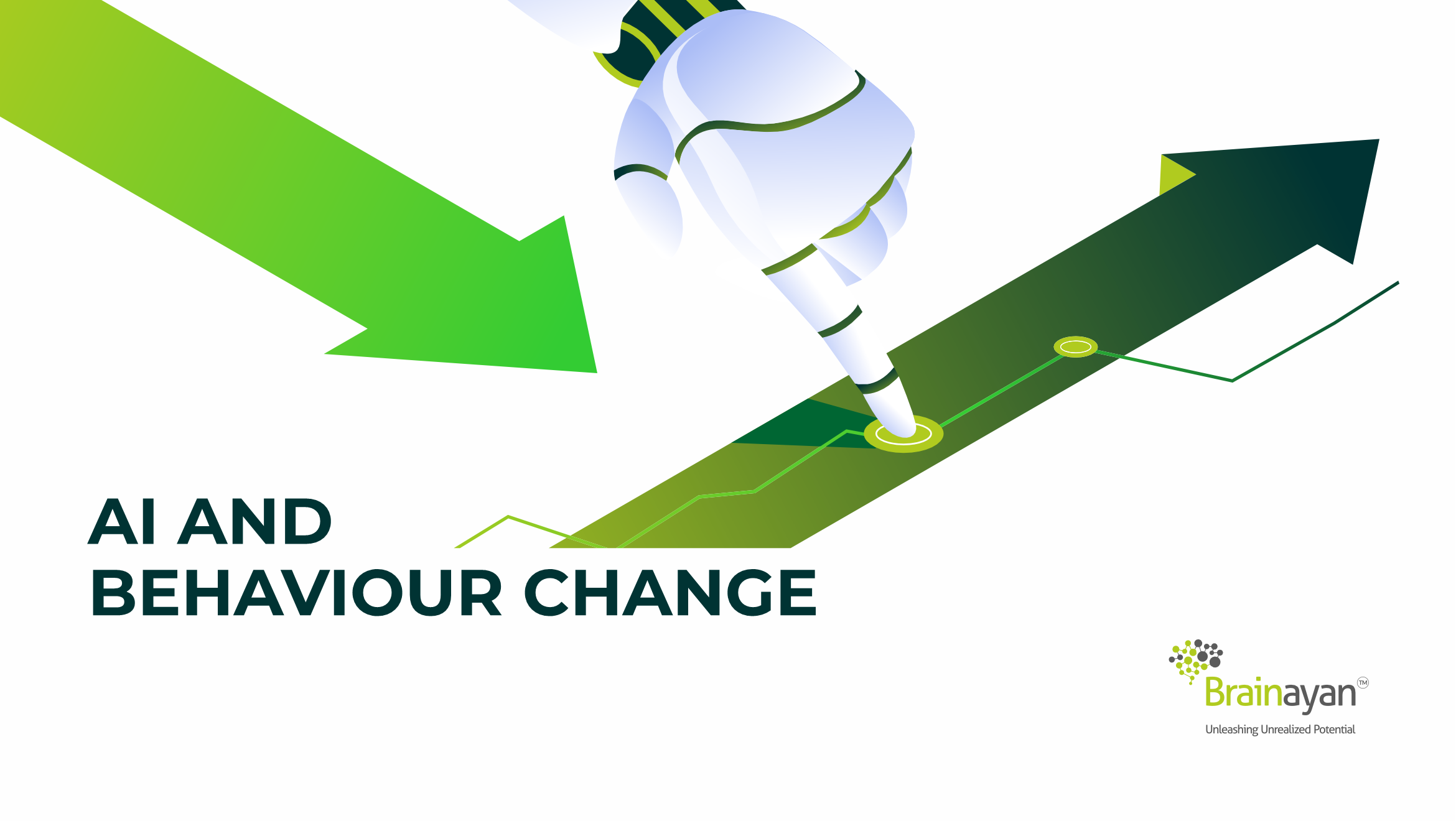
In today’s fast-paced digital world, the need for personalized experiences has never been more crucial. Traditional training methods, while highly personalized, often lacked scalability, and as technology evolved, scalability was achieved at the cost of personalization. However, with the advent of AI and machine learning, we are now able to personalize user experiences at scale. Despite this advancement, many behaviour change applications, especially in areas like learning and development and mental health, still fall short due to limitations in their conversational capabilities and reliance on static, pre-determined responses. This blog explores how AI-powered solutions, like the Manager Awesomeness App (MApp), are bridging the gap by integrating real-time, personalized coaching into everyday workflows—offering a seamless and effective way to drive behaviour change without additional effort from the user.
In general, it is observed that personalized interventions work better than the traditional generic approach [1], [2]. This is because of users finding these personalized interventions more relevant to them [3]. Functional magnetic resonance imaging (FMRI) scans confirm this hypothesis [4]. Moreover, personalization with objective data can prove to be more effective than self-reporting [5].
Most of these applications relied on chatbots with either finite states where a dialogue consists of a sequence of predetermined steps or states, or frame-based systems where a dialogue is not predetermined but dependent on the content of the user’s input and the information that the system has [6]. [7], [8]. These systems are, therefore, limited in their ability to have free conversations, mainly because of the small training data sets on human conversation in behaviour changes domains. For example, legacy apps in areas like mental health and fitness mainly used preset responses and structured question-answer formats, which lacked deep contextual understanding [9], [10]. However, generative pre-trained transformers have the capability to understand instructions and respond to the same in natural language [11]. This enables the AI models perform large number of tasks without having to be specially trained for.
Most of the modern behaviour change tools use these smart systems to process users’ data at the backend, while the users’ themselves do not get to interact with such underlying systems. For example, in tools that facilitate goal setting, interviewing skills, one-on-ones, and coaching, users interact primarily with a user-friendly interface. This limits the deliverables and user friendliness of the tool, requiring users to spend more dedicated time on the tool to feed the data in, while receiving little in return. Needless to mention the challenges associated with collecting correct data from psychometric instruments the user takes.
Manager Awesomeness App (MApp) works on the idea that aims to use the power of AI and personalization to build an environment which works proactively to get the required data from the user and maximize the deliverables. It also leverages the power of tool integration by interconnecting the data points across the features and building a comprehensive understanding of users’ context. With this approach, not only is it able to coach the users but also personalizes the responses based on user information. With an integrated learning buddy, users’ can have direct humanly interactions with the application which slips in byte-sized actionable learning during their conversation with the user. As a result, users of this application do not have to spend any extra time on learning apart from doing their work using the application. They get to learn by practicing it using the handholding of their learning buddy.
[1] Revere D, Dunbar PJ. Review of computer-generated outpa- tient health behavior interventions: clinical encounters “in absentia.”. J Am Med Inform Assoc. 2001;8(1):62-79. https:// doi.org/10.1136/jamia.2001.0080062.
[2] Aguiar M, Trujillo M, Chaves D, Álvarez R, Epelde G. MHealth apps using behavior change techniques to self-report data: sys- tematic review. JMIR MHealth UHealth. September 9 2022; 10(9):e33247. https://doi.org/10.2196/33247.
[3] Jensen JD, King AJ, Carcioppolo N, Davis L. Why are tailored messages more effective? A multiple mediation anal- ysis of a breast cancer screening intervention. J Commun. 2012;62(5):851-868. https://doi.org/10.1111/j.1460-2466. 2012.01668.x.
[4] Chua HF, Liberzon I, Welsh RC, Strecher VJ. Neural correlates of message tailoring and self-relatedness in smoking cessation programming. Biol Psychiatry. 2009;65(2):165-168. https://doi. org/10.1016/j.biopsych.2008.08.030.
[5] Tong HL, Quiroz JC, Kocaballi AB, et al. Personalized mobile technologies for lifestyle behavior change: a systematic review, meta-analysis, and meta-regression. Prev Med. 2021;148: 106532. https://doi.org/10.1016/j.ypmed.2021.106532.
[6] Hudlicka E. Virtual training and coaching of health behavior: example from mindfulness meditation training. Patient Educ Couns. 2013 Aug;92(2):160–6. doi: 10.1016/j.pec.2013.05.007. http://europepmc.org/abstract/MED/23809167. [PMC free article] [PubMed] [CrossRef] [Google Scholar]
[7] Lucas GM, Rizzo A, Gratch J, Scherer S, Stratou G, Boberg J, Morency L. Reporting Mental Health Symptoms: Breaking Down Barriers to Care with Virtual Human Interviewers. Front. Robot. AI. 2017 Oct 12;4:1–9. doi: 10.3389/frobt.2017.00051. [CrossRef] [Google Scholar]
[8] Philip P, Micoulaud-Franchi JA, Sagaspe P, Sevin ED, Olive J, Bioulac S, Sauteraud A. Virtual human as a new diagnostic tool, a proof of concept study in the field of major depressive disorders. Sci Rep. 2017 Feb 16;7(12):42656. doi: 10.1038/srep42656. doi: 10.1038/srep42656. [PMC free article] [PubMed] [CrossRef] [CrossRef] [Google Scholar]
[9] Asare, J.G. The Dark Side Of ChatGPT; Forbes: Jersey City, NJ, USA, 2023; Available online: https://www.forbes.com/sites/janicegassam/2023/01/28/the-dark-side-of-chatgpt/?sh=31f2e08a4799 (accessed on 19 February 2023).
[10] Getahun, H. ChatGPT Could Be Used for Good, But Like Many Other AI Models, It’s Rife with Racist and Discriminatory Bias. Insider. 2023. Available online: https://www.insider.com/chatgpt-is-like-many-other-ai-models-rife-with-bias-2023-1 (accessed on 19 February 2023).
[11] Radford A, Wu J, Child R, Luan D, Amodei D, Sutskever I. Language models are unsupervised multitask learners. OpenAI Blog.2019. [2020-08-29]. https://d4mucfpksywv.cloudfront.net/better-language-models/language_models_are_unsupervised_multitask_learners.pdf. [Ref list]
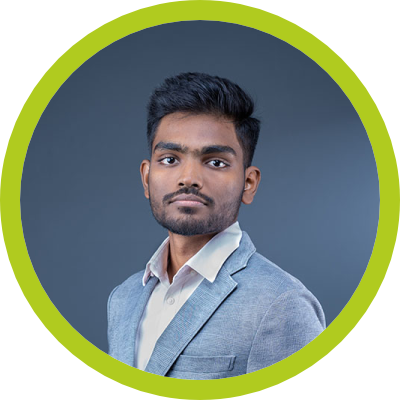
An AI Product Engineer with over two years in product development and research, Saurabh specializes in driving AI innovation and leading technical teams in startup settings. With a focus on efficient, high-impact solutions, he excels in transforming complex challenges into user-focused products that deliver measurable results.